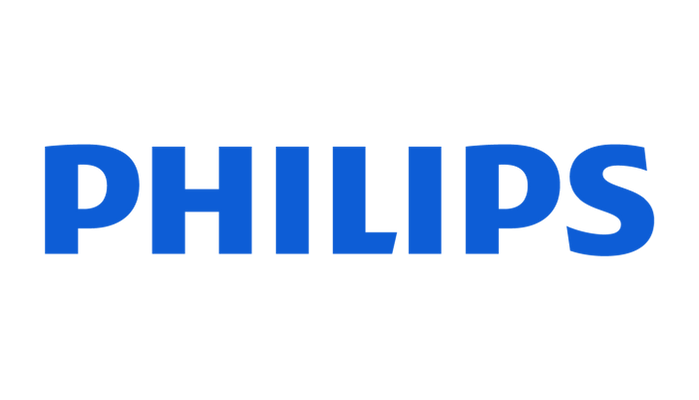
Easy Model Configuration on Low-Volume Production
This use case is geared towards utilizing techniques like simulated data, active learning, and XAI (Explainable Artificial Intelligence) to enable model training with limited training data. The incorporation of simulated data enhances the training process by exposing the model to a broad spectrum of data, even when real data is scarce. Active learning enables the selection of the most relevant training examples, increasing training process efficiency. Additionally, XAI provides insight into the model’s decision-making, which is crucial for understanding and having confidence in the results.
One of the challenges faced in the future automation of current production lines relates to flexible evaluation of product quality using visual inspection. In high-volume production environments, systems can be trained and optimized on an abundance of labeled examples, which aids the model in understanding data patterns and relationships, allowing it to generalize and make predictions on new, unlabelled data. However, due to a shift in customer demand, and the wish to be able to produce a small series of products more often, lower volume production is becoming more common. This complicates the flexibility, efficiency and scalability of production lines and does not facilitate rapid responses to product adjustments.